Around 2010, security analytics technologies started to integrate big data science and open source technologies like Hadoop (and HDFS), Pig, Mahout, etc. The goal? Ingest, process, and apply new types of algorithms to security data to supplement human intelligence for finding needles in growing haystacks of security data. The US Department of Energy was an early pioneer in this area with a project called Orca from the Oak Ridge National Lab.
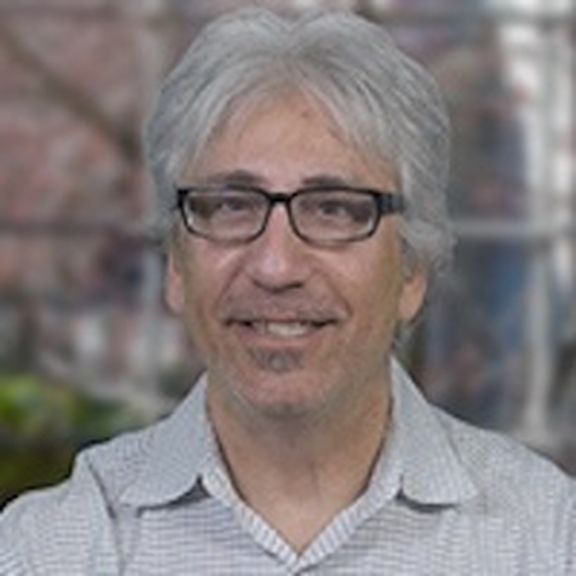
Since then, big data security analytics sort of morphed into machine learning, which led to the creation of a new security technology category, user and entity behavior analytics (UEBA).
UEBA was designed to monitor user behaviors like logins, remote access, network connections, etc., model "normal" behavior, and then detect anomalies that may indicate an attack in progress. UEBA proponents claimed that based upon this new capacity, new machine learning-based technology was destined to become a huge market as it replaced SIEM as the system of record for security analytics and operations.
When I heard the UEBA story for the first time, I had to ask a common question: Do machine learning-based security tools constitute a new type of product, or will machine learning technology simply turn into a product feature built into existing security technologies?
The market seems to have spoken. Earlier this year, RSA acquired Fortscale, a UEBA veteran. RSA plans to make Fortscale a machine learning analytics feature set for its NetWitness platform (it should also be noted that even as an independent company, Fortscale was often used as a machine learning back-end to other security technologies like authentication and DLP). Similarly, VMware scooped up E8 Security to add machine learning algorithmic muscle to its Workspace ONE modern endpoint management platform.
Those deals are far from a market anomaly; the trend has been happening for many years. Splunk acquired Caspida in 2015 to marry UEBA analytics with SIEM. HP grabbed Niara in 2017 as a security complement to Aruba network access capabilities. Bay Dynamics and Symantec have teamed up to add machine learning analytics to DLP. The list goes on and on.
It’s also worth noting that machine learning has found its way into other security technologies as a feature set. Network behavior analytics (i.e., Darktrace, Palo Alto Network’s Magnifier, Vectra Networks, etc.) all greatly leverage machine learning algorithms to spot and analyze anomalous network connections.
The same can be said for the endpoint security crowd. Traditional AV vendors like McAfee, Sophos, Symantec, Trend Micro, and Webroot have all added machine learning capabilities to bolster their ability to detect and block targeted and 0-day exploits and malware.
So, does this mean that standalone products based upon machine learning are goners? No. The intersection between artificial intelligence and security technology is still in its genesis phase and we are in a cycle of massive innovation right now, driven by cloud computing, open source, big data technologies, artificial intelligence, etc. Given this, CISOs should remain open minded about new types of more revolutionary security technologies that aren’t simple adjuncts to what they’ve done in the past.
Yup, they’ll be plenty of innovation around machine learning-based security tools, but the market is still sending a clear message to entrepreneurs and investors: Except for game-changing types of security technologies, machine learning appears to be a feature and not a product.
Jon Oltsik is an ESG senior principal analyst and the founder of the firm’s cybersecurity service. Read more ESG blogs here.